
Amanda Khatri
Editorial Manager
Unlock efficiency and compliance using AI in financial services
AI researcher and Data Scientist, Aamir Mirza, has over 10 years of experience in Machine Learning and AI in remarkably diverse environments, IoT, signal processing, NLP, NLU, Computer Vision, Finance, and Deep learning-based control systems.
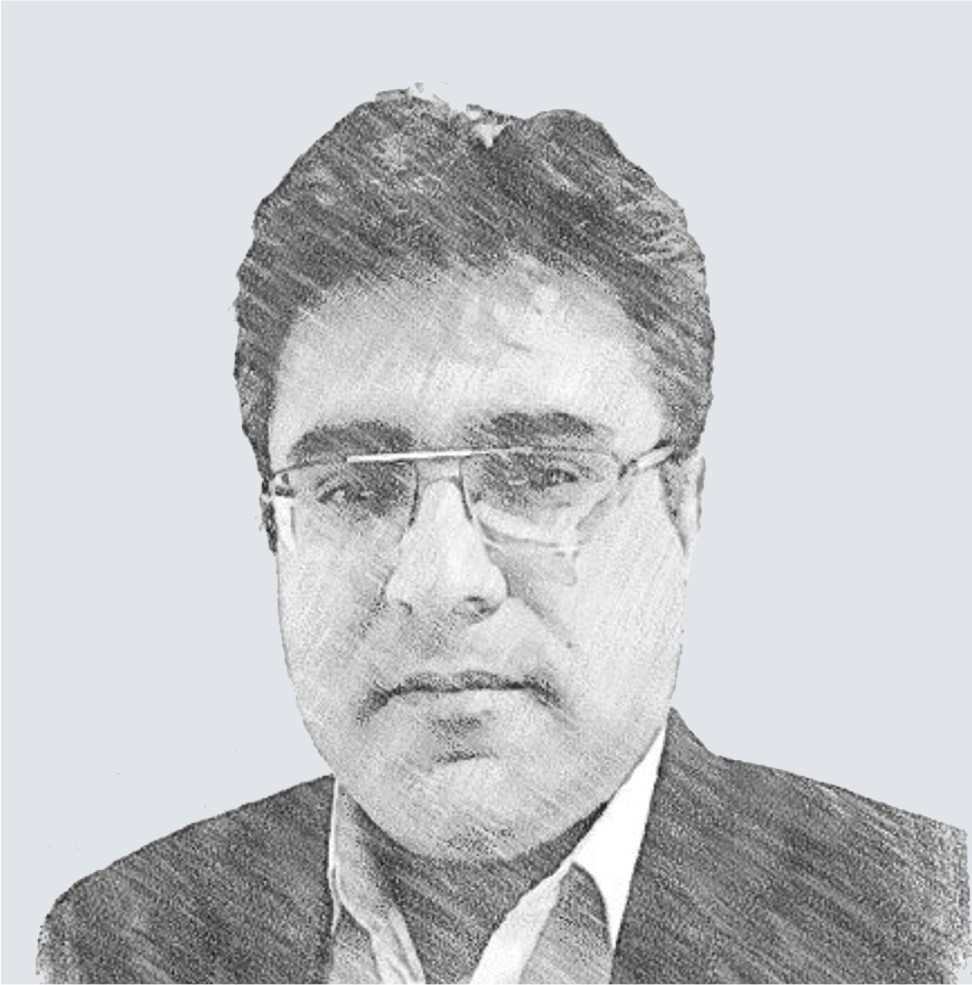
Artificial intelligence (AI) is rapidly transforming the financial services industry. From automating back-office tasks to providing personalised customer service, AI is helping financial institutions to improve efficiency, reduce costs, and increase customer satisfaction.
In the following blog post, we will demystify AI and explore its many benefits to the financial services industry and regulatory compliance as well as how AI can be used to:
- Detect fraud
- Improve decision-making
- Personalised customer service
- Comply with regulations
Additionally, the challenges associated with implementing AI in financial services will be examined, along with potential strategies for overcoming these obstacles.
Fraud detection
ML (Machine learning) methods. In financial services, fraud can be broadly classified into two sectors, compliance fraud and illegal transactions. The former is all about scammers withdrawing funds from cheques or credit accounts.
ML methods can be used to detect fraud in both compliance and illegal transactions. For compliance fraud, ML can be used to identify patterns of fraudulent activity, such as large cash deposits, transactions made from different countries or known terrorist organisations. For illegal transactions, ML can be used to identify suspicious activity, such as unauthorised transactions or unusual spending patterns.
ML methods are becoming increasingly effective at detecting fraud, and they are likely to play an even greater role in fraud detection in the future.
Here are some additional details about how ML is used to detect fraud in the finance sector.
Supervised learning
Supervised learning is a type of ML where the model is trained on a dataset of human-labelled data. This means that the model is given examples of both fraudulent and non-fraudulent transactions, and it learns to identify the patterns that distinguish between the two.
Unsupervised learning
Unsupervised learning is a type of ML where the model is not given any labelled data. This means that the model must learn to identify patterns in the data on its own. Unsupervised learning is often used to identify anomalies in data, which can be a sign of fraud. Anomalies can include Geo-spending and temporal-spending. Where does one spend money and how does one spend their money?
This technique is very popular and can be customised on a per-person basis.
Deep learning
Deep learning is a type of machine learning that uses artificial neural networks to learn from data. Neural networks are inspired by the human brain, and they can learn to identify complex patterns in data. Deep learning is often used for fraud detection because it can learn to identify patterns that would be difficult or impossible to identify using traditional methods.
ML methods are a powerful tool for detecting fraud, and they are likely to play an increasingly important role in fraud detection in the future. However, it is important to note that ML methods are not perfect, and they can sometimes generate false positives. It is vital to have a human review of any alerts generated by ML models to ensure that they are legitimate.
Improved decision making
Machine learning (ML) based methods are increasingly being used to improve the efficiency and accuracy of loan approval processes. ML algorithms can be trained on historical data to identify patterns that can be used to predict whether or not a borrower is likely to repay a loan. This information can then be used to make more informed decisions about which loans to approve and which loans to decline.
ML-based methods can also be used to automate many of the tasks involved in the loan approval process, such as data entry, risk assessment, and underwriting. This can free up human resources to focus on other tasks, such as customer service and relationship management.
As ML technology continues to develop, we can expect to see even more use of ML-based methods in loan approval processes. This will help lenders to improve the efficiency, accuracy, and profitability of their loan approval operations.
Here are some of the specific benefits of using ML-based methods for loan approval:
Improved efficiency
ML algorithms can process large amounts of data much faster than human employees. This can help to speed up the loan approval process and free up human resources for other tasks.
Improved accuracy
ML algorithms can learn to identify patterns in data that human employees may not be able to see. This can help to improve the accuracy of loan approval decisions.
Reduced costs
ML-based methods can help to reduce the costs of loan approval by automating tasks and freeing up human resources.
Overall, ML-based methods can provide several benefits for loan approval processes. By improving efficiency, and accuracy, and reducing costs, ML-based methods can help lenders to improve their bottom line.
Here are some examples of how ML-based methods are being used for loan approval:
Risk assessment
ML algorithms can be used to assess the risk of a borrower defaulting on a loan. This information can be used to determine the interest rate and terms of the loan.
Underwriting
ML algorithms can be used to underwrite loans, which means determining the amount of money that a borrower can borrow and the terms of the loan.
Data entry
ML algorithms can be used to automate data entry, which can free up human resources to focus on other tasks.
The wide adoption of ML-based methods is still in the early stages of development, but they have the potential to revolutionise the way that loan approvals are done. Gender or race bias in the underlying train dataset can lead to the marginalisation of certain communities. It is something which must be mitigated when developing supervised ML methods.
Personalised customer service
Even before Chat-GPT brought ML-based chat to the masses. Limited chat-bots were used in the banking and finance industry to take care of customer queries which were relatively simple to answer.
Deep learning-based analysis of chat logs revealed tons of valuable insights into customer interactions and where services and product offerings could potentially be improved. When it came to complex queries there was a degree of customer frustration, however those queries were handled by humans.
Post Chat-GPT, it’s looking positive for bots and customer care. Chat-GPT-type models have the potential to revolutionise the field of customer service by providing efficient and personalised assistance to customers. These models can be integrated into chatbots or virtual assistants, enabling companies to handle a large volume of customer inquiries and support requests with minimal human intervention.
Chat-GPT models excel at understanding natural language and responding contextually. They can assist customers in a wide range of tasks, including answering frequently asked questions, guiding users through troubleshooting processes, suggesting relevant products or services, and even resolving simple issues independently. By leveraging the capabilities of Chat-GPT models, businesses can enhance customer satisfaction, reduce response times, and streamline their customer service operations. These models have the potential to significantly improve the overall customer experience and drive customer loyalty.
Compliance and regulations
According to Global wire news the regulatory and compliance market is worth 1.6 billion USD as of 2021 and expected to reach 2.3 billion by 2027. CUBE is doing some exciting work by bringing ML and AI methods to this market, for example:
Summarisation
Using large language models like GPT, condense and compress large regulatory documents in the form of brief summaries which are easy to read before the end user decides on the relevance.
Effective compliance dates
One of the key questions asked around any legal compliance document is when it comes into effect and what are the dates when an organisation needs to be compliant. Since CUBE operates in every single international jurisdiction, ML-based systems are developed to operate and extract information in every single published language.
Previously this was all done manually, involving multiple researchers spanning the globe. As we transition to an autonomous system, we are freeing up internal human resources for more challenging tasks.
Citation extraction
Citations can be thought of as legal notes inserted in documents to cite which law this document refers to. CUBE has been using the ML approach to extract these citations from legal and compliance documents. Once the citation is extracted, it is then linked against multiple documents citing a common legal pattern. This helps the end user extract related documents based on common citations.
Document and structure detection
Most legal documents are distributed in either PDF or HTML format, which brings its own set of unique challenges. PDFs are compound documents, where tables, text and images exist in a single unified space. CUBE is using SOTA vision-based AI models in the domain of IDP (Intelligent document processing) to extract relevant information which is used by upstream AI models.
Watch this space as very soon there will be an in-depth examination of the ML technologies used at CUBE and their impact on regulatory compliance.
To provide any feedback or understand how CUBE uses AI, please get in touch below.